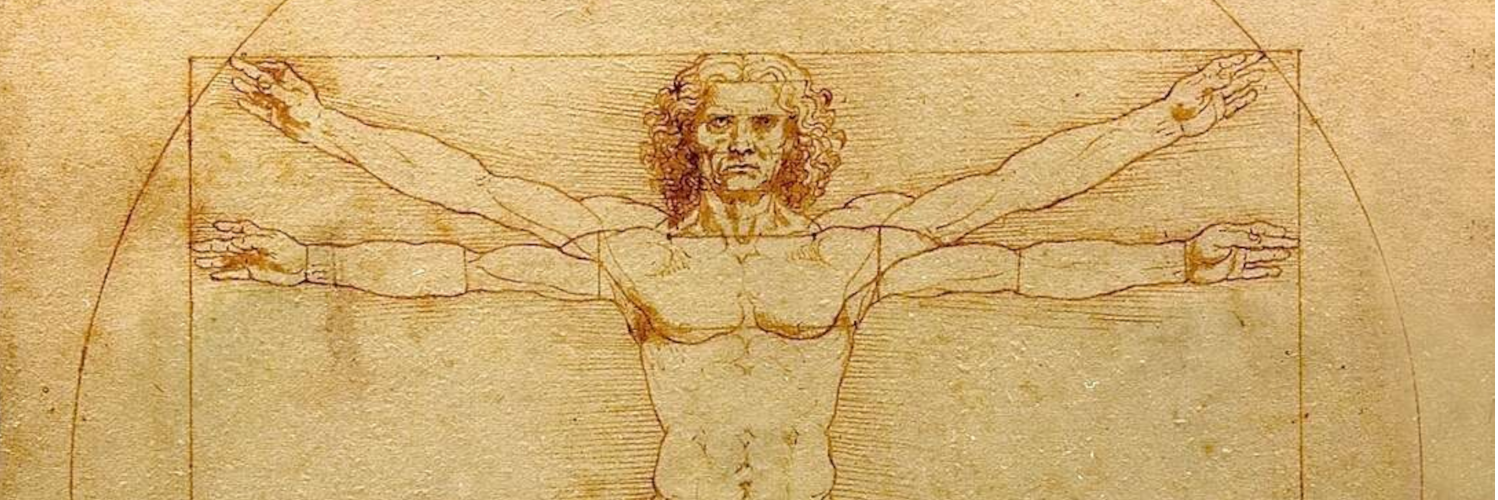
@hrefna @maegul @astrojuanlu @programming
Interesting. But seems to be limited to arrays. And I'm wondering what the benefit is over regular Numpy. In my tests I did not find Numpy to be slower than any other Blas or Lapack implementation (if type stable and immutable).
Do you have any comparisons?
In any case, I don't think it would work for solving ODEs unless I'd implement the RHS in a JIT compiled function.
I used to love Python, but now it looks like a collection of add-ons/hacks to fix the issues I have.
@maegul @astrojuanlu @programming
BTW, I'm not talking about "product code" in Julia, necessarily. The point is that I can stay at the "research code" level and don't have to rewrite anything, but can just move from a toy problem with a few parameters to a full size mod with 100s of parameters.
In my case I had a Python code solving an ODE system and a global optimisation of all parameters took a week on the 24 cores on a cluster. The same case runs over the lunch break on my laptop in Julia.
For the most recent paper a PhD student of ours use 2000-ish GPU hours for a global sensitivity/uncertainty analysis. That would have been impossible in Python or Matlab. On a single GPU that would have taken 10 years (300 years in Python), instead of 3 months*.
*yes, you can just through more GPUs at the problem, but then we can start the discussion about CO2 footprint being 40 or 600 times higher, respectively.
Note: all our benchmarks are for solving ODE system. Just doing linear algebra, there isn't really a difference in speed between Matlab, Bumpy, or Julia, because that's all going to run on the same LA libraries.
@maegul @astrojuanlu @programming
You put your finger right on it. If I tell people that they can replace Matlab or Python with Julia, then there is often not the pressure justifying learning another language.
But when I show them, that (a) the fundamental syntax is nearly the same as Matlab or (b) that the same thing in Python is much more clunky (Numpy and Scipy are not exactly elegant in my eyes), and they can get 40 and 600 times speedup respectively, then there is much more interest.
I needed the performance and DifferentialEquations.jl gave it, and my students stay for the easier language. But then they don't have a long time with Matlab or Python under their belts, so the perceived pain of learning a new language to the same level is less, of course.
At the moment we still teach Matlab mostly, because that's what employers expect, but some of my students choose Julia in addition to it. And they do like it.
More and more universities move their scientific "computational thinking" type of classes to Julia (from either Matlab or Python), which may move the trend when their will be more STEM graduates knowing the language.
Permanently Deleted
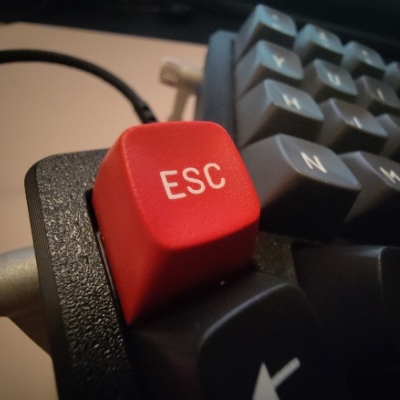
Theoretical engineer (aerospace and biomedical), associate professor of continuum mechanics, ex-petrolhead turned eco-warrior (worrier?), FOSS advocate, non-competitive cyclist, general outdoor enthusiast, renaissance man, beginner lutenist.
he/him
born@326ppm
All posts auto-delete after a while to reduce CO2 footprint.