> It feels like the US isn’t releasing what it has. I don’t think they’re behind, maybe just holding back?
The training datasets don't have the answers because the benchmark is diverse enough. That's why other models struggled to perform as well as humans until they applied the approach outlined in the paper. This is the benchmark: https://liusida.github.io/ARC/
> Test-time training (TTT) significantly enhances language models' abstract reasoning, improving accuracy up to 6x on the Abstraction and Reasoning Corpus (ARC). Key factors for successful TTT include initial fine-tuning, auxiliary tasks, and per-instance training. Applying TTT to an 8B-parameter model boosts accuracy to 53% on ARC's public validation set, nearly 25% better than previous public, neural approaches. Ensemble with recent program generation methods achieves 61.9% accuracy, matching average human scores. This suggests that, in addition to explicit symbolic search, test-time training on few-shot examples significantly improves abstract reasoning in neural language models.
A new AI model called ESM3 can generate functional proteins that would take hundreds of millions of years to evolve in nature by training on data generated by evolution.
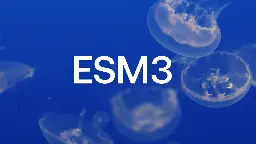
> Unlike traditional language models that only learn from textual data, ESM3 learns from discrete tokens representing the sequence, three-dimensional structure, and biological function of proteins. The model views proteins as existing in an organized space where each protein is adjacent to every other protein that differs by a single mutation event.
They used it to "evolve" a novel protein that acts similarly to others found in nature, while being structurally unique.
Top Chinese research institutions linked to the People's Liberation Army have used Meta's publicly available Llama model to develop an AI tool for potential military applications, according to three academic papers and analysts.
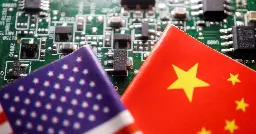
They fine-tuned a Llama 13B LLM with military specific data, and claim it works as well as GPT-4 for those tasks.
Not sure why they wouldn't use a more capable model like 405B though.
Something about this smells to me. Maybe a way to stimulate defense spending around AI?
Demand for AI offerings from Alphabet's cloud business helped drive an impressive revenue and earnings beat.
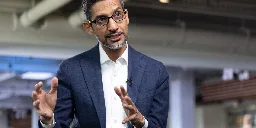
Inspired by large language models, MIT researchers developed a training technique that pools diverse data to teach robots new skills.
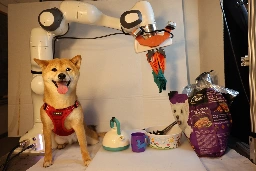
>...versatile technique that combines a huge amount of heterogeneous data from many of sources into one system that can teach any robot a wide range of tasks
>This method could be faster and less expensive than traditional techniques because it requires far fewer task-specific data. In addition, it outperformed training from scratch by more than 20 percent in simulation and real-world experiments.
Paper: https://arxiv.org/pdf/2409.20537
With the stated goal of "liberating people from repetitive labor and high-risk industries, and improving productivity levels and work efficiency"
Hopefully they can pull it off cheaply while Tesla's Optimus remains vaporware (or whatever the real world equivalent of vaporware is).